Tired of wrestling with clunky interfaces and repetitive tasks? Imagine effortlessly getting information, completing tasks, and even having meaningful conversations with your devices, just by using your voice.
We’re not quite there yet, but Natural Language Processing (NLP), a crucial component of AI, is rapidly making this a reality. This technology, often referred to as NLP in AI, is already transforming how we interact with technology, from streamlining customer service with smart chatbots to unlocking hidden insights in mountains of data.
Key Takeaways
- Natural Language Processing (NLP) is another key AI subfield that makes communication between humans and machines more intuitive. It powers intelligent machines to process human languages by combining machine learning with computational linguistics for smarter, faster language understanding.
- The NLP workflow can be described in a series of important steps, from data collection to model deployment. Each step is crucial for transforming plain text into powerful knowledge, and it flourishes on continuous improvement and partnership among multiple technologies.
- You gather open-ended text responses from citizens through comment forms, social media, and other documents. This clean, diverse, high-quality data is essential for building robust NLP models and is the foundation for successful applications.
- Tokenization and text-cleaning processes concentrate on transforming raw text into the proper format for analysis. This process raises model quality and addresses issues with thoughtful remedies, resulting in successful results.
- Numerical representation models such as Bag of Words and word embeddings convert text into numerical formats. This transformation is crucial for machine learning models, as it greatly influences the performance on various NLP tasks, and showcases the major contrast between classical and contemporary approaches.
- The possibilities that emerging trends in NLP, including conversational AI and real-time translation, will revolutionize the way we communicate and access information. This revolution is powered by new technology and ongoing research and collaboration in the field.
What is Natural Language Processing?
Natural Language Processing (NLP) is a fascinating subfield of artificial intelligence focused on enabling computers to understand and interpret human language in all its forms. This “natural language” is the everyday communication we use. NLP serves as a bridge, facilitating more natural and effective interaction between people and technology.
When you query your smartphone’s digital assistant or issue a command, you engage with NLP. This technology comprehends your spoken words and translates them into commands the computer can execute. NLP’s significance lies in its ability to enhance human-computer interactions.
By focusing on language comprehension, NLP has reshaped the technological experience, creating a more natural and inclusive interaction point. At its heart, NLP combines machine learning with computational linguistics—the study of using algorithms and models to understand and generate language data.
This powerful fusion allows machines not only to grasp human language but also to produce contextually relevant responses. With roots in linguistics and a history spanning over 50 years, NLP constantly evolves with new technological developments.
One of NLP’s greatest strengths is its ability to blend various computational techniques. This capability allows machines to analyze, understand, and generate human language more effectively.
Using syntax, NLP derives meaning from language based on grammatical rules, enabling computers to comprehend natural language and humans do.
This forms the foundation for everyday applications like search engines, voice-activated navigation systems, and smart assistants such as Amazon’s Alexa, Apple’s Siri, and Microsoft’s Cortana. These tools rely on NLP to operate seamlessly, creating a more natural, human, and efficient technology experience.
NLP’s impact on our tech-driven lives is profound. Chatbots that handle basic customer service inquiries are at the forefront of this trend. With voice-powered systems, machines engage in real-time with spoken commands. Beyond chatbots, NLP facilitates advanced data processing, automating functions like customer service, data analysis, and document management.
How NLP Works
Natural Language Processing (NLP) is a critical component of modern artificial intelligence, transforming the intricacies of human language into meaningful insights. Its workflow follows a series of stages, from data collection to model deployment.
Text Input and Collection
The NLP process begins with gathering text data from diverse sources, such as social media, documents, and user interactions. This diversity ensures models are robust and adaptable across various contexts.
A comprehensive dataset that considers all aspects of an issue improves the training process, leading to more accurate and reliable NLP models. Methods for collecting high-quality text data are crucial, providing the groundwork for successful NLP applications.
Data Preprocessing Steps
Preprocessing converts raw text into an analyzable format. Key steps include:
- Tokenization
- Stop Word Removal
- Stemming
- Text Cleaning
Free Tips on Humanizing AI Content

Make your AI content sound human-like

Bypass AI detectors

Humanizing prompts
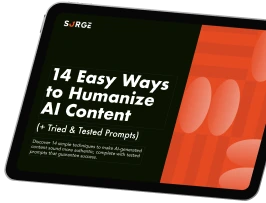

These processes are essential for data cleansing, enhancing NLP model performance by improving precision. NLP faces challenges like processing misspellings and understanding different dialects, but targeted strategies can effectively address these issues.
Text Representation Techniques
Converting text into numerical formats is crucial for machine learning models to understand language data. Techniques such as Bag of Words and word embeddings serve this purpose.
The chosen representation significantly impacts performance. Newer methods, like embeddings, enhance performance by better capturing underlying semantic relationships in the text.
Feature Extraction Methods
Feature extraction identifies the most critical information from text data. Techniques like the term frequency-inverse document frequency (TF-IDF) and n-grams are widely used.
Selecting interpretable features is essential for boosting model performance. This process converts unstructured text into organized, standardized information, making it easier to analyze and understand.
Choosing and Training Models
Selecting the best model involves considering specific NLP tasks. Supervised learning, where models are trained on labeled datasets, is central to this process. Hyperparameter tuning optimizes performance, while ongoing evaluation allows models to adapt and improve based on new data inputs. This iterative process is fundamental to NLP’s success.
Deployment and Inference Process
Deploying NLP models requires additional steps, like developing APIs for easy integration. Once deployed, models use the inference process to predict outcomes on new data. Monitoring ensures models adapt to new information, maintaining accurate and effective results.
Evaluation and Optimization Practices
Key evaluation metrics include:
- Accuracy
- Precision
- Recall
- F1 Score
These metrics assess model performance on held-out test datasets. While optimization techniques improve efficiency, continuous user feedback provides real-world insights to further refine models, ensuring they meet practical demands.
Continuous Improvement Strategies
NLP technology must adapt to reflect the evolving nature of language. Continual updates and user feedback are vital for this adaptation. Retraining new data keeps models current and relevant, and ongoing research drives rapid advancements in NLP technologies.
Future of Natural Language Processing
Natural Language Processing (NLP) stands on the brink of a paradigm shift, driven by powerful forces and accelerating technology. These emerging trends are poised to transform our interaction with machines and how we consume information.
Emerging Trends in NLP
- Conversational AI
- Real-time Language Translation
- Personalized Content Generation
Free Tips on Humanizing AI Content

Make your AI content sound human-like

Bypass AI detectors

Humanizing prompts
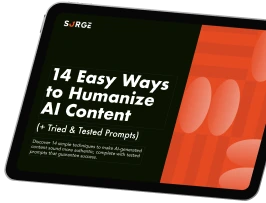

These converging trends offer profound opportunities for both industries and individuals. Conversational AI, a leading trend in technology, is revolutionizing customer service by enabling companies to provide swift, tailored responses. This trend improves both consumer experience and operational efficiency.
Real-time language translation breaks down language barriers, allowing for seamless conversations worldwide. Similarly, personalized content generation helps businesses create content that deeply resonates with users, boosting engagement and satisfaction.
NLP’s potential to fundamentally alter how we communicate and access information is evident. It also benefits e-commerce companies through sentiment analysis, which provides a deep understanding of customer sentiment and preferences by processing vast amounts of data.
This NLP application lets businesses base decisions on customer needs, improving satisfaction and loyalty. These trends are fostering the development of innovative NLP applications. For instance, NLP models are now used to provide stock recommendations and financial insights by analyzing large datasets to deliver actionable advice.
Potential Enhancements
Future advancements in NLP are largely centered on context and emotion. These innovations will shape the future of human-computer interaction, creating a more natural and empathetic experience.
Combining NLP with machine learning and computer vision opens up incredible possibilities, producing holistic solutions that address complex challenges. For instance, integrating NLP with advancements in AI chips allows models to handle more parameters, making them more capable.
Advancements in computational power have also played a crucial role, particularly in developing sophisticated NLP models. As discussed last year, AI chip makers are pushing the boundaries of processing power, enabling NLP models to train on larger datasets and support more complex functionalities.
The expanding NLP market, with North America holding the largest market share, underpins this growth. Collaboration among researchers, developers, and industry professionals is essential to drive the NLP industry forward.
Continuous research and innovation are necessary to address ethical challenges, ensuring discoveries and technologies reflect societal values. AI ethics will guide the future of NLP technologies, steering the responsible development and deployment of these powerful tools.
Frequently Asked Questions
What are the benefits of NLP in AI?
It brings significant improvements to AI by allowing machines to communicate more effectively with humans. It also automates tedious language-based tasks, enhances customer service, and reveals valuable insights from text data. It’s the same technology that drives smart voice assistants and translation services.
What is the future of NLP?
The future of NLP looks bright. This is where advances in AI and machine learning will allow us to create a much more accurate, intuitive, and context-aware understanding of language. This will add new capabilities to apps like chatbots, translation, and sentiment analysis.
Why is NLP important in AI?
NLP is important since it serves as the critical junction between human communication and computer comprehension. This is what enables AI to understand and generate language, making technology more conversational and human-like.
How is NLP used in everyday life?
NLP is the technology behind smart voice assistants like Siri and Alexa. It’s the same technology powering your chatbots, translation apps, and sentiment analysis tools. It also powers more human customer support automation and native user experiences across multiple customer-facing applications.
What challenges does NLP face?
NLP still struggles with the nuances of human language, such as understanding context, sarcasm, and various dialects. It also takes a hit in terms of language ambiguity and cultural nuances. Continued research is focusing on these challenges to enable deeper language understanding.